Learn how to use the empirical rule (or 68-95-99.7 rule) to estimate probabilities for normal distributions in statistics. From Ramanujan to calculus co-creator Gottfried Leibniz, many of the world's best and brightest mathematical minds have belonged to autodidacts. And, thanks to the Internet, it's easier than ever to follow in their footsteps (or just finish your homework or study for that next big test). With this installment from Internet pedagogical superstar Salman Khan's series of free math tutorials, you'll learn how to estimate probabilities for a bell distribution.
Home
Math How to Estimate probabilities with the empirical rule
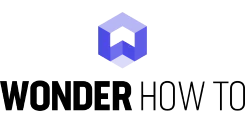
By getexcellent
May 25, 2010 11:06 PM
The next big software update for iPhone is coming sometime in April and will include a Food section in Apple News+, an easy-to-miss new Ambient Music app, Priority Notifications thanks to Apple Intelligence, and updates to apps like Mail, Photos, Podcasts, and Safari. See what else is coming to your iPhone with the iOS 18.4 update.
Comments
Be the first, drop a comment!